1, 2, 3, 4, 5, 6, 7, 8 AND 9 THESE NUMBERS ARE THE BUILDING BLOCKS OF YOUR PIN CODE. YOUR DATE OF BIRTH DEFINES YOUR CHARACTER.Everything has a meaning in life. We come to life, grow up, and when the time comes we leave this earth. Would you like to question the meanings of the numbers this time? Let’s start with calculating your pin code.
Every individual has an 8 digit pin code. The ninth number that we come up with adding these numbers together gives us our life sense number. Do not underestimate those numbers. Each of them presents you clues about your character, your lack of relationships, your position in your business life, and your dreams for the future. The only thing you need to do is to calculate the number as explained.
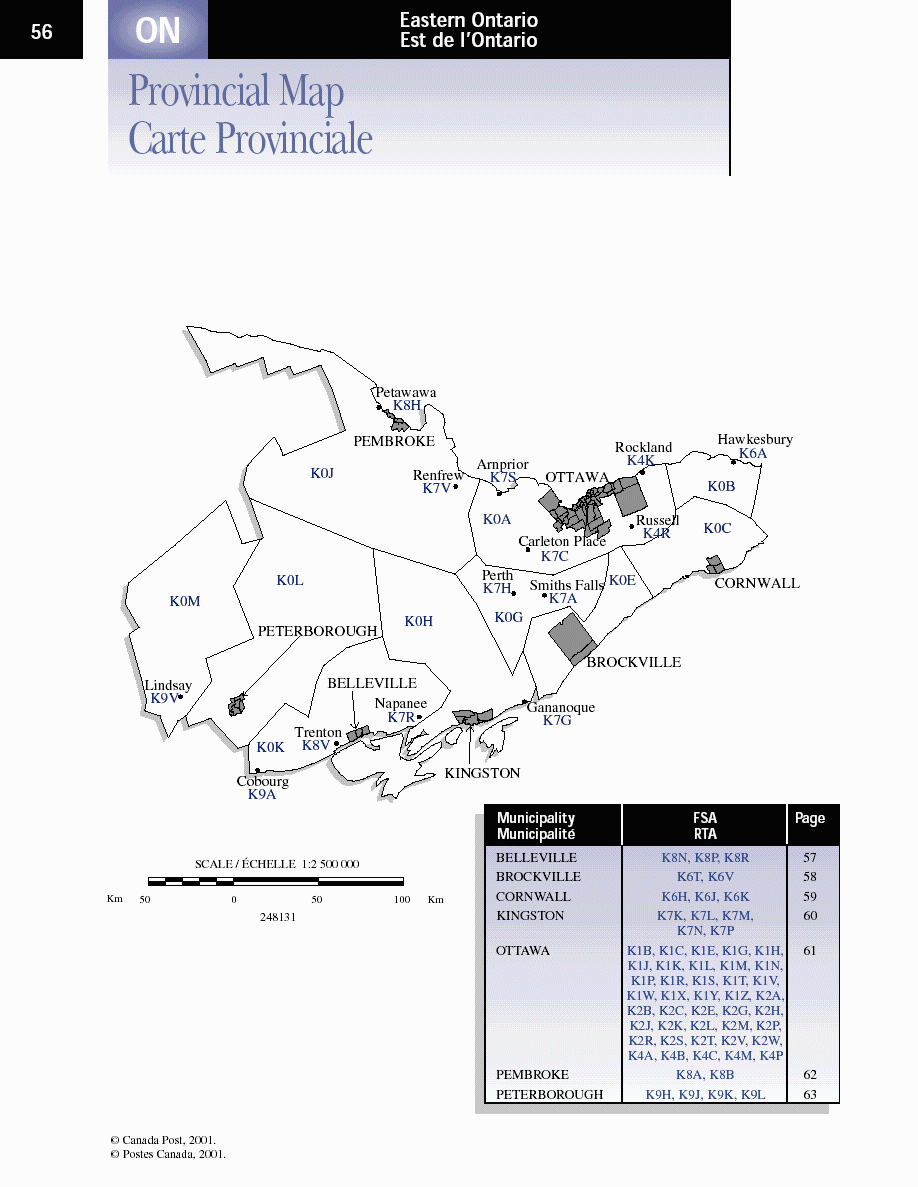
The results are going to surprise you as much as they surprised us.Douglas Forbes, the author of the book named ‘Human Pin Code’, says that he had tested the code on over 25 thousand people in 25 years. Forbes makes remarks about human pin code: “Your pin code reveals your strengths and weaknesses. The sacred formula of your faith and your date of birth presents you a different guide map. Every day is a matrix by itself and your date of birth is the key to that matrix. A 9 digit matrix is created based on your birth day, birth month, and birth year.

The sum of the first 8 digits gives you your life sense number. When the 9 digits are deciphered, the matrix gives us information about the operating systems that define the dynamics of human character. Number 1 reflects visual-memory; number 2: hearing-sense, number 3: taste-urge, number 4: smell-being practical, number 5: touch-memory, number 6: sense-urge, number 7: spirit-sense, number 8: creation-being practical, and number 9 reflects life with all of its values.”Sample pin code analysis: 45115966How do you calculate your pin code?
First, draw 9 squares on a piece of paper. Let’s say your date of birth is May 13, 1972.
You should place 13 in the first square. But you can only work with one digit. Therefore, you cannot place 13 in the first square as it is. The sum of 1 and 3 goes into your first square: 4. Put your month of birth into the second square. May is the fifth month, so the number 5 goes into the second square. If your month of birth was December, you were going to have to place 1+2=3 into the second square.
The sum of the numerals in your year of birth goes into the third square. 1+9+7+2 makes 19.
And 1+9 makes 10. So, you need to place 1+0=1 into the third one. To fill the fourth square sum up the values in the first three squares.
4+5+1 makes 10, so 1+0 makes 1 that goes into the fourth square. For the fifth square, you need to sum up the values of the first and the fourth square, so 1+4= 5 goes into the fifth square. To fill the sixth square, sum up the values of the first and the second squares. 4+5=9 goes into the sixth square. To find the seventh number sum up the values of the second and the third squares. 5+1=6 goes into the seventh square.The meanings of the numbersTo calculate the eighth value, sum up the sixth and the seventh numbers. 9+6=15 and 1+5 makes 6.
Therefore, 6 goes into the eighth square. To find your life sense number add all of the 8 numbers together. 4+5+1+1+5+9+6+6 makes 37. 3+7=10 and that gives us 1. That goes into the last square. It is that simple.
Each number has a special meaning. For example, number 1 reflects the people who are creative, fun, outgoing, proud, self-confident leaders. Number 2 represents the people with extraordinary imaginations, who value parenting and have strong instincts to protect their families and loved ones.
Number 3 expresses a military manner and sets an example to productiveness and order. Number 4 represents honesty, righteousness, integrity. We can tell that you are an adrenalin addict if you have 5s mostly. Number 6 identifies with people who are friendly, compassionate, romantic, quiet, and coherent. 7 identifies with majors that require artistic creativeness such as advertising, acting, painting, sculpting. Number 8 represents people with quiet nature, and who are methodic and patient.
9 reflects intelligent, childish, and artistic spirits, pure minds, innocence.
. 726 Downloads.AbstractWe revisit the side-channel attacks with brain–computer interfaces (BCIs) first put forward by Martinovic et al. At the USENIX 2012 Security Symposium. For this purpose, we propose a comprehensive investigation of concrete adversaries trying to extract a PIN code from electroencephalogram signals.
Overall, our results confirm the possibility of partial PIN recovery with high probability of success in a more quantified manner and at the same time put forward the challenges of full/systematic PIN recovery. They also highlight that the attack complexities can significantly vary in function of the adversarial capabilities (e.g., supervised/profiled vs. Unsupervised/non-profiled), hence leading to an interesting trade-off between their efficiency and practical relevance. We then show that similar attack techniques can be used to threat the privacy of BCI users. We finally use our experiments to discuss the impact of such attacks for the security and privacy of BCI applications at large, and the important emerging societal challenges they raise. State of the art The increasing deployment of Brain–computer interfaces (BCIs) allowing to control devices based on cerebral activity has been a permanent trend over the last decade. While originally specialized to the medical domain (e.g., , ), such interfaces can now be found in a variety of applications.
Notorious examples include drowsiness estimation for safety driving and gaming. Quite naturally, these new capabilities come with new security and privacy issues, since the signals BCIs exploit can generally be used to extract various types of sensitive information ,. For example, at the USENIX 2012 Security Symposium, Martinovic et al. Showed empirical evidence that electroencephalogram (EEG) signals can be exploited in simple, yet effective attacks to (partially) extract private information such as credit card numbers, PIN codes, dates of birth and locations of residence from users. These impressive results leveraged a broad literature in neuroscience, which established the possibility to extract such private information (e.g., see for lie detection and for neural markers of religious convictions). Or less invasively, they can be connected to linguistic research on the reactions of the brain to semantic associations and incongruities (e.g., , ). All these threats are gaining relevance with the availability of EEG-based gaming devices to a general public ,.
In our experiments, eight people (next denoted as users) agreed to provide the 4-digit PIN code that they consider the most significant to them, meaning the one they use the most frequently in their daily life. This PIN code was given by the users before the experiment started, stored during the experiment and deleted afterward for confidentiality reasons. Five other random 4-digit codes were generated for each user (meaning a total of six 4-digit codes per user).Each (real or random) PIN was then shown on a computer exactly 150 times to each user (in a random order), meaning a total of 900 events for which we recorded the EEG signal in sets of 300, together with a tag T ranging from 1 to 6 (with (T=1) the correct PIN and (T=2) to 6 the incorrect ones).
We used 32 Ag–AgCl electrodes for the EEG signals collection. These were placed on the scalp using a WaveGuard cap from Cephalon, using the international 10-10 system. The stimulus onset asynchrony (SOA) was set to 1.009 s (i.e., slightly more than 1 s, to reduce the environmental noise). The time each PIN was shown was set to 0.5 s. When no PIN was displayed on the screen, a + sign was maintained in order to keep the focus of the user on the center of the screen. We additionally ensured that two identical 4-digit codes were always separated by at least two other 4-digit codes. The split of our experiments in sub-experiments of 300 events was motivated by a maximum duration of 5 min, during which we assumed the users to remain focused on the screen.
This MATLAB R2015a is a complete simulation package for engineering applications which deal with designing of electrical and mechanical control systems. MathWorks MATLAB R2015a OverviewMathWorks MATLAB R2015a is a high-level language and interactive environment for numerical computation, visualization, and programming of electrical and mechanical control systems. Download MathWorks MATLAB R2015a free setup for windows. Mathworks matlab r2015a 64 bit. It offers an intuitive and graphical user interface for analyzing data, developing algorithms, and creating models and applications.
The signals were amplified and sampled at a 1000 Hz rate with a 32-channel ASA-LAB EEG system from Advanced NeuroTechnologies. Eventually, and in order to identify eye blinks which potentially perturb the EEG signal, we added two bipolar surface electrodes on the upper left and lower right sides of the right eye and rejected the records for which such an artifact was observed.
This slightly reduced the total number of events stored for each user. (Precisely, this number was reduced to 900, 818, 853, 870, 892, 887, 878, 884, for users 1–8.)This simplified setting naturally comes with limitations.
First and concretely, the number of possible PIN codes for a typical smart card would of course be much larger than the 6 ones we investigate (e.g., 10,000 for a 4-digit PIN). In this respect, we first insist that the primary goal of the following experiments is to investigate the information leakages in EEG signals thoroughly, and this limited number of PIN codes allowed us to draw conclusions with good statistical confidence. Yet, we also note that this setting could be extended to a reasonable threat model. For example, one could target (approx 1000) different users by repeatedly showing them (approx 10) PIN codes among the 10,000 possible ones and recover one PIN with good confidence. Second, and since the attacks we carry out essentially test familiar versus unfamiliar information, there is also a risk of false positives (e.g., an all zero code or a close to correct code). In this respect, our mitigation plan is to exploit statistical tools minimizing the number of false negatives, therefore potentially allowing enumeration among the most likely candidates.
3 Methodology.From a practical point of view, building a model for all the PINs and users seems impractical in real-world settings: this would require being able to collect multiple observations for each of the 10,000 possible values of a 4-digit code. Furthermore, and as discussed in Sect., our real versus random profiling allowed us to lean toward realistic (non-profiled) attacks.From a neurophysiological point of view, the information we aim to extract is based on event-related potentials (ERPs) that have been shown to reflect semantic associations and incongruities ,. In this respect, while we can expect a user to react differently to real and random 4-digit codes, there is no reason for him to treat the random codes differently. (Up to problems due to the apparition of other “significant” values that may lead to false positives, as will be discussed next.)The scheme of Fig. Represents the general procedure we followed to analyze our EEG data (similar to side-channel analysis). We next detail its main steps. Selection of electrodes As mentioned in introduction, each original observation is made of 32 vectors of 1000 samples, leading to a large amount of data to process.
To simplify our treatments, we started by analyzing the different electrodes independently. Among the 32 ones of our cap, Electrodes P7, P8, Pz, O1 and O2 gave rise to non-negligible signal (see Fig.
), which is consistent with the existing literature where ERPs related to semantic associations and incongruities were exhibited in the central/parietal zones ,. Our following analyses are based on the exploitation of the Electrodes P7 and P8 which provided the most regular information across the different users. For illustration, Figs. And represent the mean and standard deviation traces corresponding to two different users. (Similar figures for the other users are available in appendices, as shown in Figs., and Figs.,.) From these examples, a couple of relevant observations can already be extracted (and will be useful for the design and interpretation of our following evaluations). First, we see (on the left parts of Fig. ) that the EEG signals may be more or less informative depending on the users and electrodes.
More precisely, we generally noticed informative ERP components after 300–600 ms (known as the P300 component) for most users and electrodes, which is again consistent with the existing literature ,. Yet, our measurements also put forward user-specific differences in the shape of the mean traces corresponding to the correct PIN value.
(Note that the figures mostly show examples of informative EEG signals, but for one user and some other electrodes, no such clear patterns appear.) Second, and quite importantly, the difference between the left and right parts of the figures illustrates the significant gain when moving from an unsupervised/unprofiled evaluation context to a supervised/profiled one. That is, while in the first case, we need the traces corresponding to the correct PIN value to stand out, in the second case, we only need it to behave differently than the others. 4Exemplary standard deviation traces for different tag values corresponding to User 8, Electrode P7 (left) and User 6, Electrode P7 (right)Eventually, a look at the standard deviation curves in Fig. Suggests that the measurements are quite noisy, hence non-trivial to exploit with a limited amount of observations. This will be confirmed in our following PDF estimation phase and therefore motivates the dimensionality reduction in the next section (intuitively because using more dimensions can possibly lead to better signal extraction, which can mitigate the effect of a large noise level).Dimensionality reduction The evaluation of our metrics requires to build a probabilistic model, which may become data intensive as the number of dimensions in the observations increases. For example, directly estimating a 2000-dimensional PDF corresponding to our selected electrodes is not possible. In order to deal with this problem, we follow the standard approach of reducing dimensionality.
More precisely, we use the principal component analysis (PCA) that was shown to provide excellent results in the context of side-channel attacks against cryptographic devices. We investigate two options in this direction.The value of the PI estimated using the maximum profiling set (i.e., the extreme right values in the graphs). It reflects the informativeness of the model built in the profiling phases and is correlated with the success rate of the online (maximum likelihood) attack using this model.
Positive PI values indicate that the model is sound (up to Footnote 5) and should lead to successful online attacks if the number of observations (i.e., the q parameter in our notations) used by the adversary is sufficient.The number of traces in the profiling set required to reach a positive PI. It reflects the (offline) complexity of the model estimation (profiling) phase. 7Evolution of the PI in function of the size of the profiling set for Users 3 (top) and 6 (bottom), using average PCA (left) and raw PCA (right)In this respect, the results in Fig. Show a positive convergence for the two illustrated users, yet toward different PI values which indicate that the informativeness of the EEG signals differs between them.
Next, and quite interestingly, we also see that the difference between average PCA (in the left part of the figure) and raw PCA (in the right side) confirms the expected intuitions. Namely, the fact that raw PCA reduces dimensionality based on less meaningful criteria and requires more dimensions implies a slower model convergence. Typically, model convergence was observed in the 100 observations’ range with average PCA and required up to 400 traces with raw PCA.
For completeness, Table contains the estimated PI values with maximum profiling set, for the different users and types of PCA. Excepted for one user (User 5) for which we could never reach a positive PI value with confidence, this analysis suggests that all the users lead to exploitable information and confirms the advantage of average PCA. A similar table obtained with the Gaussian profiling is given in Appendix. We now move to the more challenging problem of unsupervised/non-profiled attacks. For this purpose, we first applied the attack sketched in Sect. With the maximum number of traces in the profiling set.
That is, we repeated our evaluation of the PI metric six times, assuming each of the tag values to be the real one. Furthermore, we computed the confidence intervals for each of the PI estimates according to the confidence paragraph in the previous section. The results of this experiment are in Fig. For two users and lead to three observations.
10Confidence intervals for the (non-profiled) PI evaluation of Sect. With (approx 900) observations (top), (approx 450) observations (middle) and (approx 225) observations (bottom), for Users 8 (left) and 6 (right)First, looking at the first line of the figure, which corresponds to the correct PIN value, we can now confirm that the PI estimates of Sect.
Are sufficiently accurate (e.g., the confidence intervals clearly guarantee a positive PI). Second, the confidence intervals for the random PIN values (i.e., tags 2–6) confirm the observation from our success rate curves (Fig. ) that the users react similarly to all random values. Third, the middle and bottom parts of the figure show the results of two (resp. 4) non-profiled attacks where the profiling set was split in 2 (resp.
4) independent parts (without resampling), therefore leading to the evaluation of 2 (resp. 4) confidence intervals for each tag value. Concretely, the PI estimate for the correct PIN value consistently started to overlap with the ones of random PINs for all users, as soon as the number of attack traces q was below 200, and no clear gain for the correct PIN could be noticed below (q=100). This confirms the intuition that unsupervised/non-profiled side-channel attacks are generally more challenging than supervised/profiled ones (here, by an approximate factor 5–10 depending on the users).This conclusion also nicely matches the one in Sect., Fig., where we already observed that the (offline) estimation of an informative model is more expensive than its (online) exploitation for PIN code recovery as measured by the success rate and average rank (by similar factors). Indeed, in the unsupervised/non-profiled context such an estimation has to be performed “on-the-fly”. 4.3 Model portabilitySince the previous section suggests a significant advantage of supervised/profiled attacks over unsupervised/non-profiled ones, a natural question is whether the profiling can lead to realistic attack models. Clearly, estimating a model for the correct PIN of each user an adversary would like to target seems hardly realistic (especially if 10,000 PIN values are considered).
Therefore, and in order to get around this drawback, a solution would be to use the model built for one user against another user. Despite limited by the number of users in our experiments, we made preliminary analyses in this direction. Interestingly, while for most pairs of users the resulting attacks failed and the PI estimates remained negative, we also found two pairs of users for which the models could be mutually exchanged. Namely, targeting User 1 (resp. User 6) with the model of User 6 (resp. User 1) leads to a PI of 0.0211 (resp.
Human Pin Code Wikipedia
And targeting User 1 (resp. User 3) with the model of User 3 (resp. User 1) leads to a PI of 0.0281 (resp. Intuitively, this positive result is in part explained by the similar shapes of the first eigenvectors used to reduce the dimensionality when estimating these models.
Overall, this problem of model portability is in fact similar to the problem of variability faced in the context of side-channel attacks against cryptographic devices. Hence, it is an interesting scope for further research to investigate how advanced profiling techniques (e.g., profiling multiple users jointly with mixture models) could be used to increase the practical relevance of supervised/profiled attacks against the human brain.
Note that in this context, the impact of certain parameters in our methodology is susceptible to evolve too. For example, and as just mentioned, the user specificities that make the portability of the models challenging are in part due to the shape of the eigenvectors produced by the average PCA. So using the raw PCA may gain interest in this case. As a preliminary experiment in this direction, we evaluated the PI when targeting a user with a model profiled with all the other users. As a result, we could obtain positive PI values for 5 out of 7 users, with both the average and the raw PCA (and similar informativeness). For illustration, the success rate curves for such a (successful and unsuccessful) profiling are given in Fig.
These results suggest that profiling classes of similar users is certainly a promising approach for realistic attacks. The results in this paper lead to two conclusions.First, and from the security point of view, our experiments show that PIN extraction attacks using BCIs are feasible, yet require several observations to succeed with high probability. In this respect, the difference between the complexity of successful supervised/profiled attacks (around 10 correct PIN observations) and unsupervised/non-profiled attacks (more in the hundreds range) is noticeable.
It suggests the aggregation of users into classes for which the models are sufficiently similar as an interesting scope for further research (which would require larger scale experiments, with more users). In this setting, a better investigation of the impact of enumeration would also be worthwhile. Indeed, the reduction of the average rank of the correct PIN is also significant in our analyses. Therefore, combining side-channel attacks against the human brain with some enumeration power can reduce the number of observations required to succeed. (Roughly, we can assume that the average key rank will be reduced exponentially in the number of observations, as usually observed in side-channel attacks.)More generally, our results suggest that extracting concrete PIN codes from EEG signals, while theoretically feasible and potentially damaging from some users and PINs, is not yet a very critical threat for systematic PIN extraction. This may change in the future, if/when massive amounts of BCI signals start to be collected.
Besides, other targets with smaller cardinality could already be more worrying (e.g., extracting the knowledge of one relative among a set of unknown people displayed on a screen), because of avoiding issues related to users loosing their focus due to too long experiments.Second, and given the importance of profiling for efficient information extraction from EEG signals, our experiments also underline that privacy issues may be even more worrying than security ones in BCI-based applications. Indeed, when it comes to privacy, the adversary trying to identify a user is much less limited in his profiling abilities. In fact, any correlation between his target user and some feature found in a dataset is potentially exploitable. Furthermore, the amount and types of correlations that can be exhibited in this case are potentially unbounded, which makes the associated risks very hard to quantify. In this respect, the data minimization principle does not seem to be a sufficient answer: it may very well be that the EEG signals collected for one (e.g., gaming) activity can be used to reveal various other types of (e.g., medical, political) correlations. Anonymity is probably not the right answer either (since correlations with groups of users may be as discriminant as personal ones). And such issues are naturally amplified in case of malicious applications (e.g., it seems possible to design a BCI-based game where situations lead the users to incidentally reveal preferences).
So overall, it appears as an important challenge to design tools that provide evidence of “fair treatment” when manipulating sensitive data such as EEG signals, which can be connected to emerging challenges related to computations on encrypted data which can be connected to emerging challenges related to computations on encrypted data.